E-commerce Product Recommendation Dataset: Analyzing Data Sources
- Data as a Service (DaaS) Software Marketing & Analytics
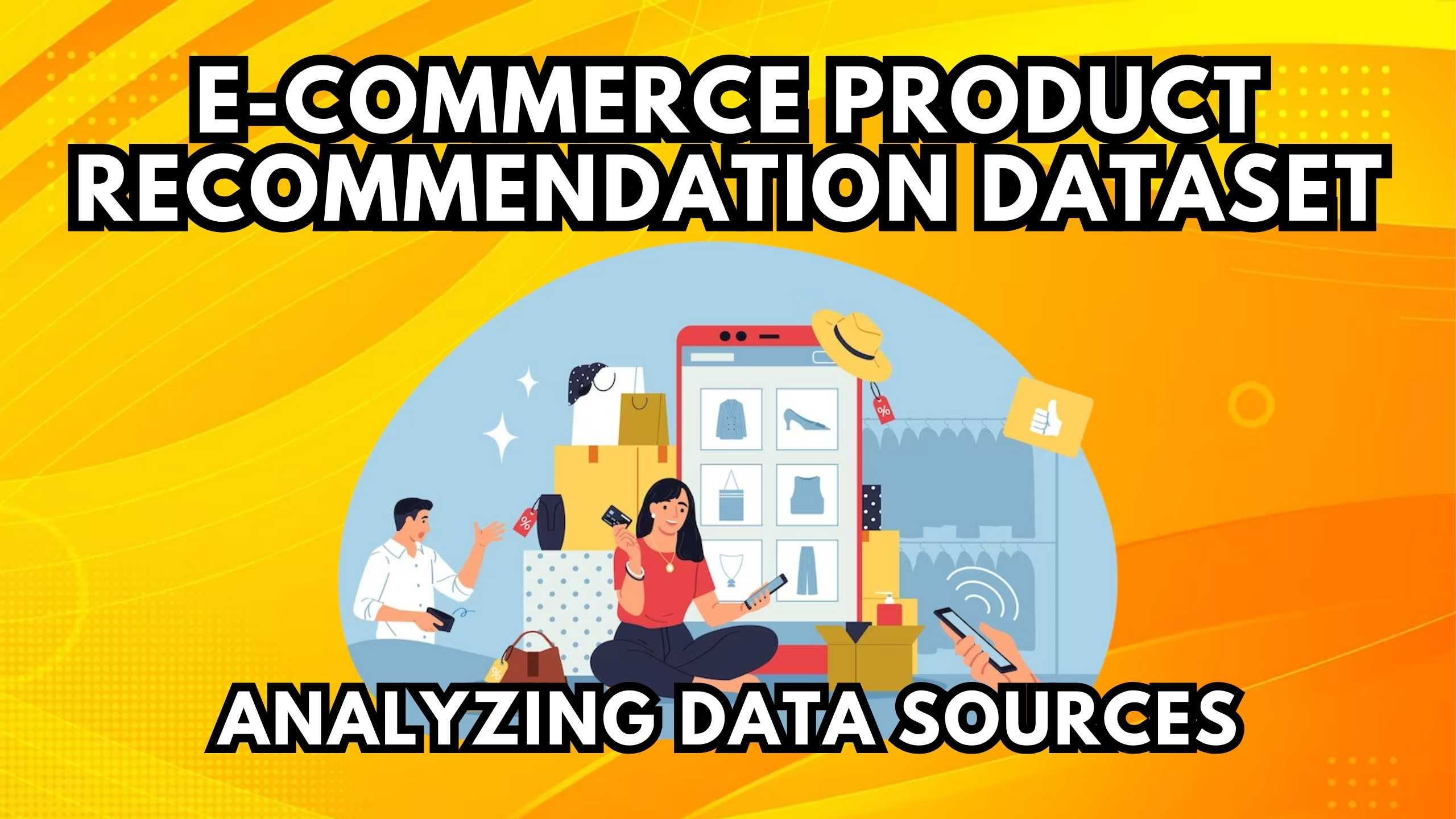
E-commerce Product Recommendation Dataset: Analyzing Data Sources
In the dynamic landscape of e-commerce, the effectiveness of product recommendation systems relies heavily on robust datasets. This blog delves into the intricate world of e-commerce product recommendation datasets, exploring the diverse sources that contribute to the creation of intelligent and personalized recommendation algorithms.
1. User Interaction Data: The Foundation of Personalization
At the core of any e-commerce recommendation system is user interaction data. Platforms like Amazon gather immense amounts of data on user behavior, including clicks, views, purchases, and time spent on product pages. Analyzing this data provides insights into user preferences, helping to create personalized recommendations that enhance the overall shopping experience.
For businesses venturing into e-commerce, understanding and leveraging user interaction data is pivotal to developing effective recommendation algorithms.
2. Demographic and Geographic Data: Tailoring Recommendations to Users
Demographic and geographic data play a crucial role in refining product recommendations. Platforms like Sephora use customer demographics to offer beauty product suggestions tailored to individual preferences and regional trends. This level of personalization increases the likelihood of user engagement and conversion.
E-commerce businesses can benefit from incorporating demographic and geographic data to ensure their recommendations resonate with the unique characteristics of their target audience.
3. Purchase History: Predicting Future Preferences
Analyzing the purchase history of users is a potent strategy employed by Netflix in the entertainment industry. By understanding past choices, e-commerce platforms can predict future preferences and offer product recommendations aligned with users’ evolving tastes. This not only fosters customer loyalty but also drives additional sales.
For businesses, leveraging purchase history data enhances the accuracy of product recommendations, providing users with items they are more likely to purchase.
4. Social Media Signals: Amplifying Personalization
Social media signals, as seen in platforms like Pinterest, offer valuable insights into users’ interests and trends. Integrating social media data into recommendation algorithms allows e-commerce businesses to amplify personalization by suggesting products that align with users’ broader preferences and lifestyle.
Understanding and harnessing social media signals can elevate the effectiveness of recommendation engines, enhancing user satisfaction and engagement.
5. Feedback and Reviews: Refining Recommendation Accuracy
Platforms like TripAdvisor leverage user feedback and reviews to enhance their recommendation systems for travel-related products. Incorporating customer reviews and feedback into datasets refines the accuracy of recommendations, providing users with insights from real experiences.
For e-commerce businesses, actively seeking and incorporating customer feedback can contribute to the continual improvement of recommendation algorithms.
Relevant SaaS Products:
- Amazon Personalize: Leverage the power of Amazon’s recommendation engine to enhance user engagement and drive sales by incorporating personalized product recommendations based on user behavior.
- Sisense: Analyze and visualize diverse datasets, including user interactions and demographic information, to extract meaningful insights and optimize your e-commerce recommendation strategies.
- Segment: Streamline the collection and utilization of customer data from various sources, including social media signals, to create a unified view for personalized recommendations.
- Yotpo: Enhance your recommendation algorithms by incorporating customer feedback and reviews into the dataset, providing valuable insights to refine product suggestions.
- Recombee: Utilize a comprehensive recommendation API to integrate personalized product suggestions based on diverse data sources, optimizing user experiences on your e-commerce platform.
Conclusion
In conclusion, e-commerce product recommendation datasets are a multifaceted amalgamation of user interactions, demographics, purchase history, social media signals, and feedback. As businesses navigate the intricacies of these datasets, they unlock the potential to deliver hyper-personalized recommendations, fostering customer satisfaction and loyalty.
Elevate Your E-commerce Strategy with Subscribed.fyi!
Ready to enhance your e-commerce strategy? Subscribed.fyi provides exclusive deals on essential SaaS tools. Sign up for free to unlock secret deals and access savings on data analytics tools and other resources. Optimize your recommendation algorithms, boost user engagement, and stay ahead in the competitive e-commerce landscape with Subscribed.fyi.
Relevant Links:
- Amazon Personalize
- Sisense
- Segment
- Yotpo
- Recombee
- Amazon
- Sephora
- Netflix
- TripAdvisor
- Subscribed.fyi