Exploring Personalized Recommendation Systems
- AI Writing Assistant Popular Tools AI Tools
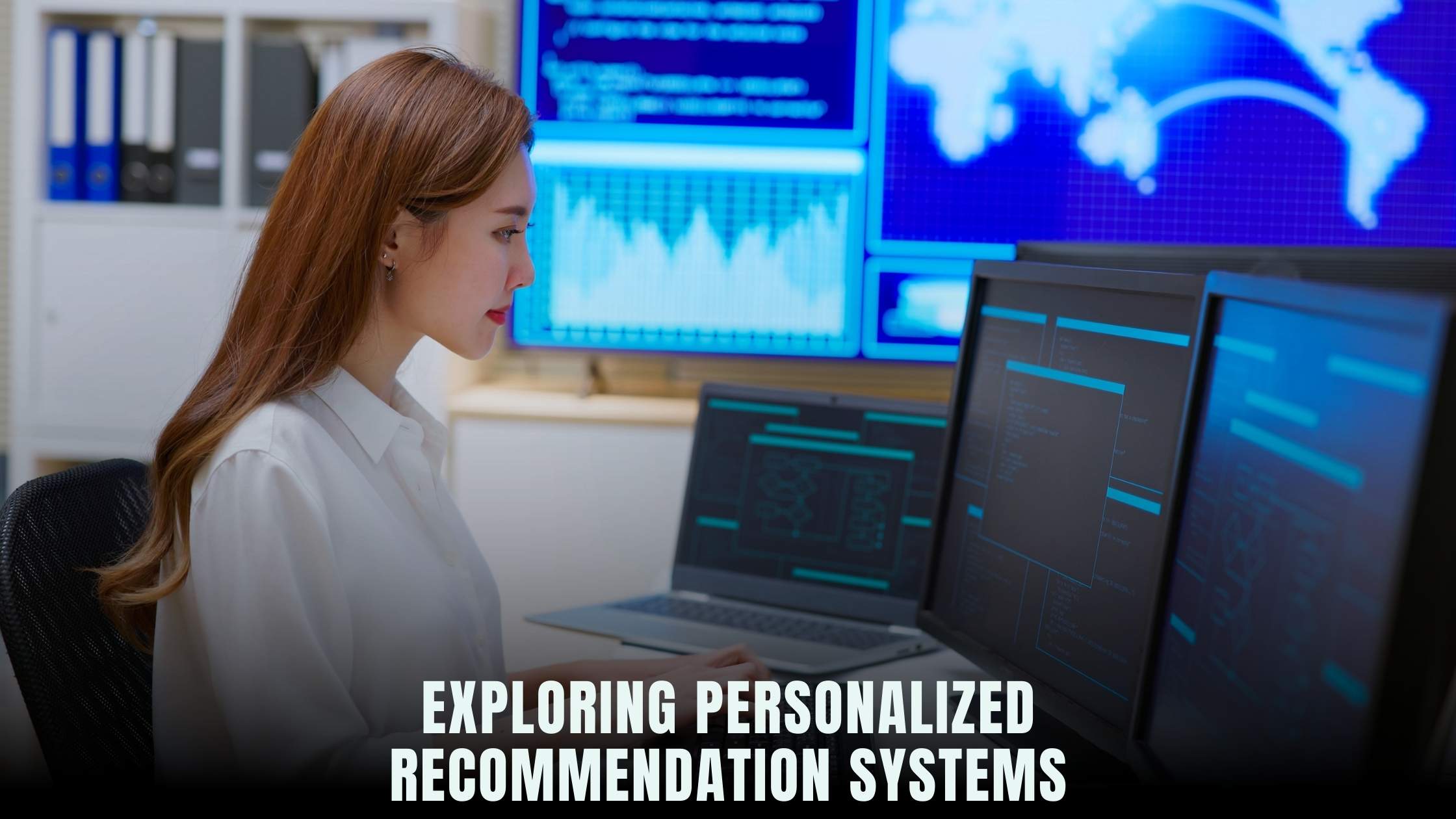
Exploring Personalized Recommendation Systems
In the era of digital transformation, personalized recommendation systems have become integral to enhancing user experiences across various online platforms. These systems leverage advanced algorithms and user data to deliver tailored recommendations, driving engagement, and satisfaction. In this article, we’ll delve into the intricacies of personalized recommendation systems, their key features, and how businesses can leverage them to optimize their online offerings.
Understanding Personalized Recommendation Systems
Personalized recommendation systems are algorithmic tools designed to analyze user preferences, behaviors, and interactions to deliver tailored suggestions. Whether it’s recommending products on e-commerce websites, movies on streaming platforms, or articles on news websites, these systems aim to provide users with relevant content based on their interests and past interactions.
Key Components of Personalized Recommendation Systems:
- User Profiling: Personalized recommendation systems create user profiles by analyzing historical data such as browsing history, purchase behavior, and demographic information. These profiles help in understanding individual preferences and interests.
- Content Analysis: These systems employ content analysis techniques to extract features and attributes from items in the inventory, such as products, movies, or articles. By understanding the characteristics of each item, the system can make accurate recommendations.
- Collaborative Filtering: Collaborative filtering is a common approach used in recommendation systems, where user preferences are inferred from similar users’ behavior. This technique identifies patterns and similarities among users to generate recommendations.
- Machine Learning Algorithms: Machine learning algorithms play a crucial role in personalized recommendation systems, as they continuously learn and adapt based on user feedback. These algorithms improve recommendation accuracy over time by adjusting to changing user preferences.
- Real-Time Recommendation: With the increasing volume of data and user interactions, personalized recommendation systems must be capable of delivering real-time recommendations. This requires efficient processing and analysis of data to provide timely suggestions to users.
Key Features of Personalized Recommendation Systems
Let’s explore some key features that make personalized recommendation systems effective:
1. Personalization:
- Personalized recommendation systems tailor recommendations to each user’s unique preferences and behavior.
- By analyzing historical data and user interactions, these systems can predict what items a user is likely to be interested in, enhancing user engagement and satisfaction.
2. Recommendation Diversity:
- Effective recommendation systems strive to provide diverse recommendations that cater to different user interests and preferences.
- By offering a variety of recommendations, these systems ensure that users are exposed to a wide range of content and products, increasing the likelihood of user engagement and conversion.
3. Contextual Relevance:
- Personalized recommendation systems take into account the context in which recommendations are made, such as the user’s current activity, location, and device.
- By considering contextual factors, these systems can deliver more relevant and timely recommendations, enhancing the overall user experience.
4. Scalability:
- As user bases and data volumes grow, personalized recommendation systems must be scalable to handle increased computational demands.
- Scalable architecture enables these systems to process and analyze large datasets efficiently, ensuring that recommendations remain accurate and timely even as the user base expands.
5. User Feedback Mechanisms:
- To improve recommendation accuracy, personalized recommendation systems often incorporate user feedback mechanisms.
- Users can provide explicit feedback by rating or liking recommended items, as well as implicit feedback through their interactions and engagement with the recommendations.
Relevant SaaS Products for Personalized Recommendation Systems
When it comes to implementing personalized recommendation systems, several SaaS products offer robust solutions tailored to the needs of businesses. Here are some notable examples:
- RichRelevance: RichRelevance offers a comprehensive personalization platform that includes product recommendations, content personalization, and search optimization solutions.
- Evergage: Evergage provides real-time personalization and customer data platform solutions, allowing businesses to deliver personalized experiences across channels.
- Dynamic Yield: Dynamic Yield offers a personalization platform that enables businesses to deliver individualized experiences, including recommendations, product discovery, and messaging.
- Sailthru: Sailthru provides a customer engagement platform that includes personalized email marketing, website personalization, and mobile marketing solutions.
- Monetate: Monetate offers a personalization platform that helps businesses deliver personalized experiences across channels, including websites, mobile apps, and email.
Leveraging Subscribed.FYI for Personalized Recommendation System Solutions
For businesses seeking to implement or enhance their personalized recommendation system capabilities, Subscribed.FYI provides valuable insights and resources. With a curated selection of SaaS products and tools tailored to the needs of businesses, Subscribed.FYI empowers users to discover, evaluate, and select the best recommendation system solutions for their specific requirements.
Unlock exclusive deals and savings on premium recommendation system solutions with Subscribed.FYI Deals, and take your personalization efforts to the next level.
Relevant Product Links: