Ferret: Apple’s Precision Language Model for Spatial Understanding
- AI Image Generators Software AI Writing Assistant Popular Tools AI Tools
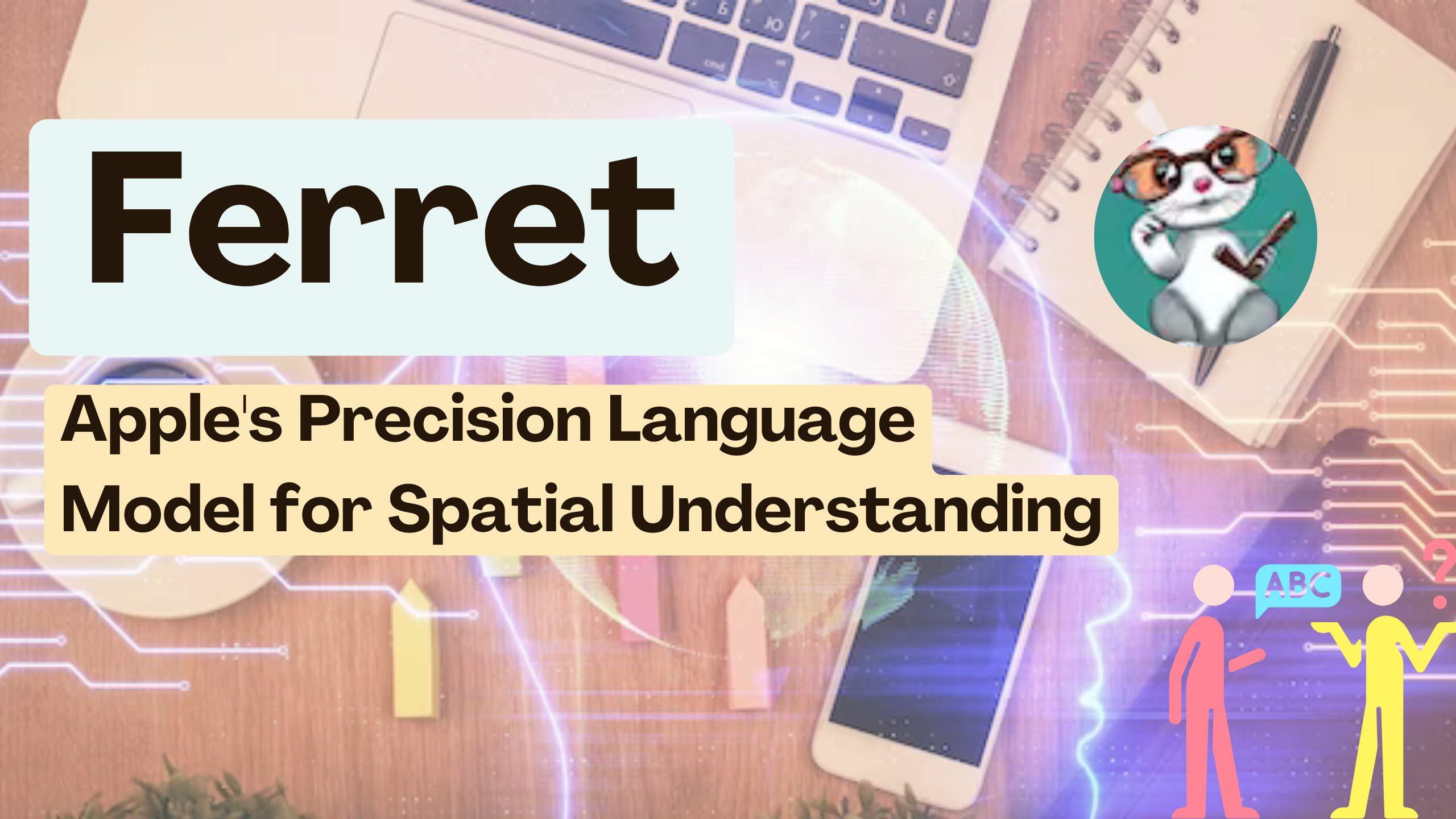
Ferret: Apple’s Precision Language Model for Spatial Understanding
In the ever-evolving landscape of language models, Apple introduces a groundbreaking advancement with Ferret. This Multimodal Large Language Model (MLLM) is designed to excel in both image understanding and language processing, showcasing remarkable proficiency in comprehending spatial references. This article delves into the intricacies of Ferret, exploring its unique features and contributions to spatial understanding.
Unveiling Ferret: A Multimodal Marvel
Understanding Spatial References
Ferret represents a leap forward in MLLM technology by introducing a novel approach to understanding spatial references. It boasts the capability to refer and ground anything within an image, irrespective of shape or granularity. The key lies in Ferret’s hybrid region representation, a powerful amalgamation of discrete coordinates and continuous features. This integration allows Ferret to represent regions in images with unparalleled accuracy.
Spatial-Aware Visual Sampler
To extract continuous features from diverse regions, Ferret incorporates a spatial-aware visual sampler. This innovative component is adept at handling varying sparsity across different shapes, enabling Ferret to accept a wide range of region inputs, including points, bounding boxes, and free-form shapes. The result is a language model that can seamlessly understand and process complex spatial information.
GRIT Dataset: A Comprehensive Instruction Tuning Dataset
To enhance Ferret’s capabilities, Apple curated the GRIT dataset, comprising approximately 1.1 million samples. This large-scale, hierarchical dataset provides rich spatial knowledge, including 95,000 hard negative data samples to promote the robustness of the model. The GRIT dataset serves as a crucial component in fine-tuning Ferret for superior performance in referring and grounding tasks.
Key Contributions
- Ferret Model: The hybrid region representation and spatial-aware visual sampler enable fine-grained and open-vocabulary referring and grounding in MLLM.
- GRIT Dataset (~1.1M): A large-scale, hierarchical, robust ground-and-refer instruction tuning dataset.
- Ferret-Bench: A multimodal evaluation benchmark that jointly requires Referring/Grounding, Semantics, Knowledge, and Reasoning.
Exploring Ferret’s Capabilities
Beyond its contributions, Ferret stands out in various evaluations, showcasing superiority in classical referring and grounding tasks. It outperforms existing MLLMs in region-based and localization-demanded multimodal chatting. The evaluations also reveal a significantly improved capability of describing image details and a remarkable alleviation in object hallucination.
Conclusion
As we witness the continuous evolution of language models, Ferret emerges as a precision tool for spatial understanding, redefining the boundaries of what is achievable. Apple’s commitment to innovation is evident in Ferret’s hybrid approach and the curated GRIT dataset, laying the foundation for advancements in multimodal language processing.
Embark on the journey of spatial understanding with Ferret – where language meets precision in the world of images. While you explore the frontiers of language models, don’t forget to check out other groundbreaking technologies like Subscribed.FYI, a platform revolutionizing subscription management for SMBs and SaaS providers.
Accessing Ferret Resources
Explore more about Ferret and its groundbreaking technology through the following official links:
- Ferret on Product Hunt
- Ferret on arXiv
- Ferret Code and Data on GitHub
- For additional inquiries or information, you can reach out to Apple’s Research Team.
- Subscribed.FYI – Streamlining subscription management for SMBs and SaaS providers.