Hybrid Recommendation System: Blending Approaches for Optimal Results
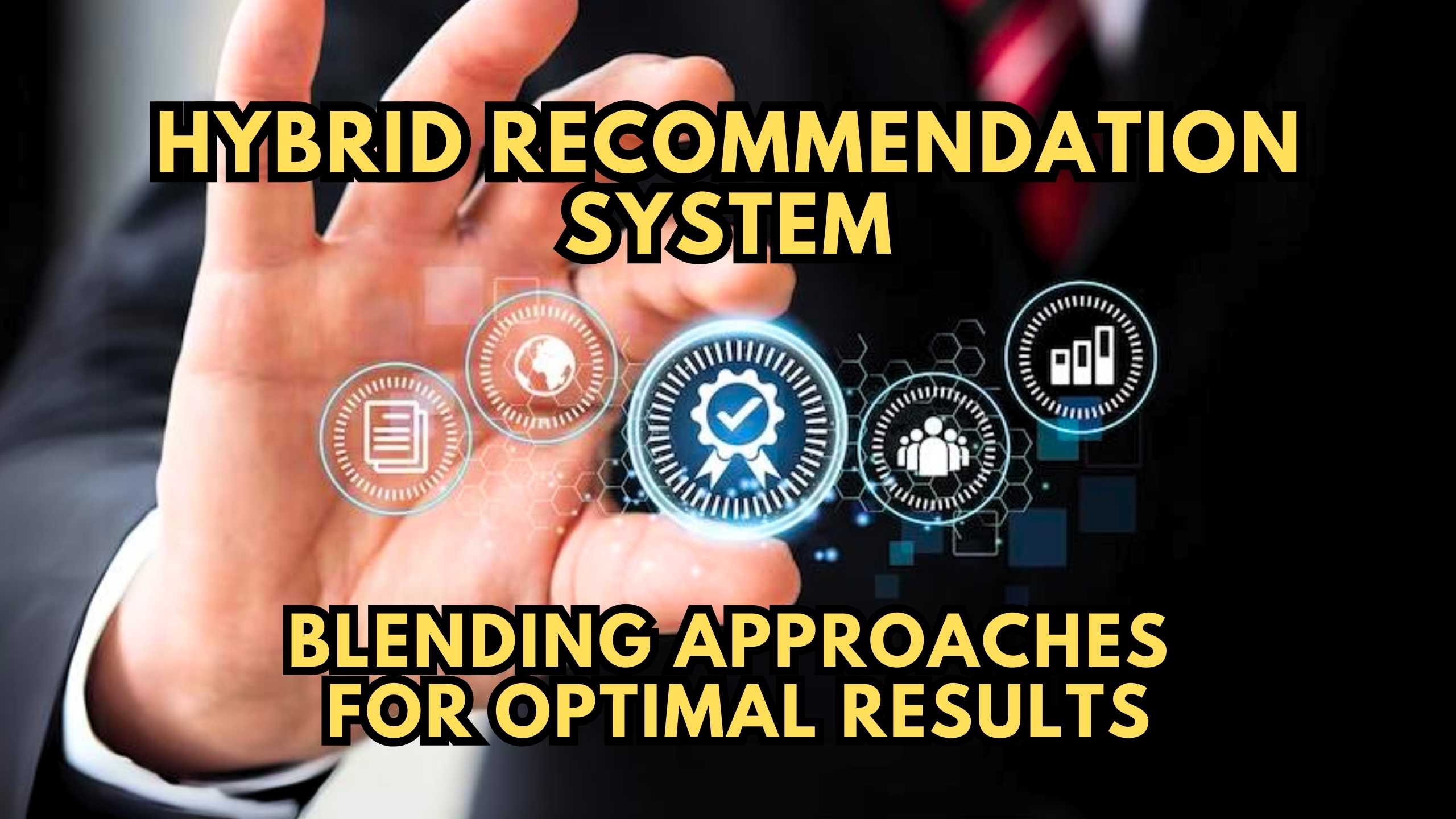
Hybrid Recommendation System: Blending Approaches for Optimal Results
In the ever-evolving landscape of recommendation systems, the hybrid approach has emerged as a game-changer. By seamlessly integrating different recommendation techniques, businesses can achieve optimal results, providing users with a personalized experience that transcends the limitations of singular methodologies. Let’s delve into the intricacies of hybrid recommendation systems and explore the relevant SaaS products that can enhance their implementation.
Unveiling the Essence of Hybrid Recommendation Systems
A hybrid recommendation system is a sophisticated solution that combines multiple recommendation approaches to provide users with more accurate and diverse suggestions. These approaches typically include collaborative filtering, content-based filtering, and sometimes, knowledge-based methods. By leveraging the strengths of various models, hybrid systems overcome individual limitations and deliver enhanced user experiences.
Navigating the Landscape of Recommendation Techniques
- Collaborative Filtering: This technique relies on user behavior and preferences. By analyzing patterns and similarities among users, collaborative filtering recommends items that like-minded users have appreciated. The challenge lies in handling sparse data and the cold start problem.
- Content-Based Filtering: Content-based filtering suggests items based on the attributes of the items and the user’s preferences. It’s particularly effective in addressing the cold start problem, where there is limited user interaction data. However, it may struggle to discover new and unexpected items for users.
- Knowledge-Based Methods: These methods recommend items based on explicit knowledge about users and items. While they can be powerful in specific domains, they may lack scalability and struggle with keeping up with rapidly changing user preferences.
Enhancing Hybrid Recommendations with SaaS Solutions
- Segment: Segment provides a robust customer data platform, enabling businesses to collect and utilize data effectively. Integrating Segment into a hybrid recommendation system ensures a seamless flow of user interaction data for collaborative filtering.
- Optimizely: Optimizely’s experimentation and personalization solutions can be invaluable for testing and optimizing different recommendation algorithms. This iterative approach is essential in refining the hybrid model for optimal performance.
- Zendesk: Zendesk’s customer support platform can complement a hybrid recommendation system by incorporating user feedback and preferences into the recommendation algorithms, improving the overall accuracy of suggestions.
- Crazy Egg: Crazy Egg’s analytics tools, such as heatmaps and user recordings, provide insights into user behavior. This data is valuable for content-based filtering, allowing businesses to understand how users interact with items and tailor recommendations accordingly.
- Marketing Cloud: Marketing Cloud offers real-time personalization solutions, enhancing the dynamic nature of hybrid recommendation systems. The ability to adapt recommendations based on user interactions in real-time contributes to a more responsive and effective system.
Conclusion
In conclusion, a hybrid recommendation system represents the epitome of personalized user experiences. By judiciously combining collaborative filtering, content-based filtering, and knowledge-based methods, businesses can offer recommendations that resonate with users across diverse scenarios. To embark on the journey of implementing a powerful recommendation system and discover exclusive deals on essential SaaS tools, sign up for free on Subscribed.fyi Deals.
Relevant Links: