E-commerce Recommendation System Research Paper: Investigating Innovations
- Data as a Service (DaaS) Software Marketing & Analytics
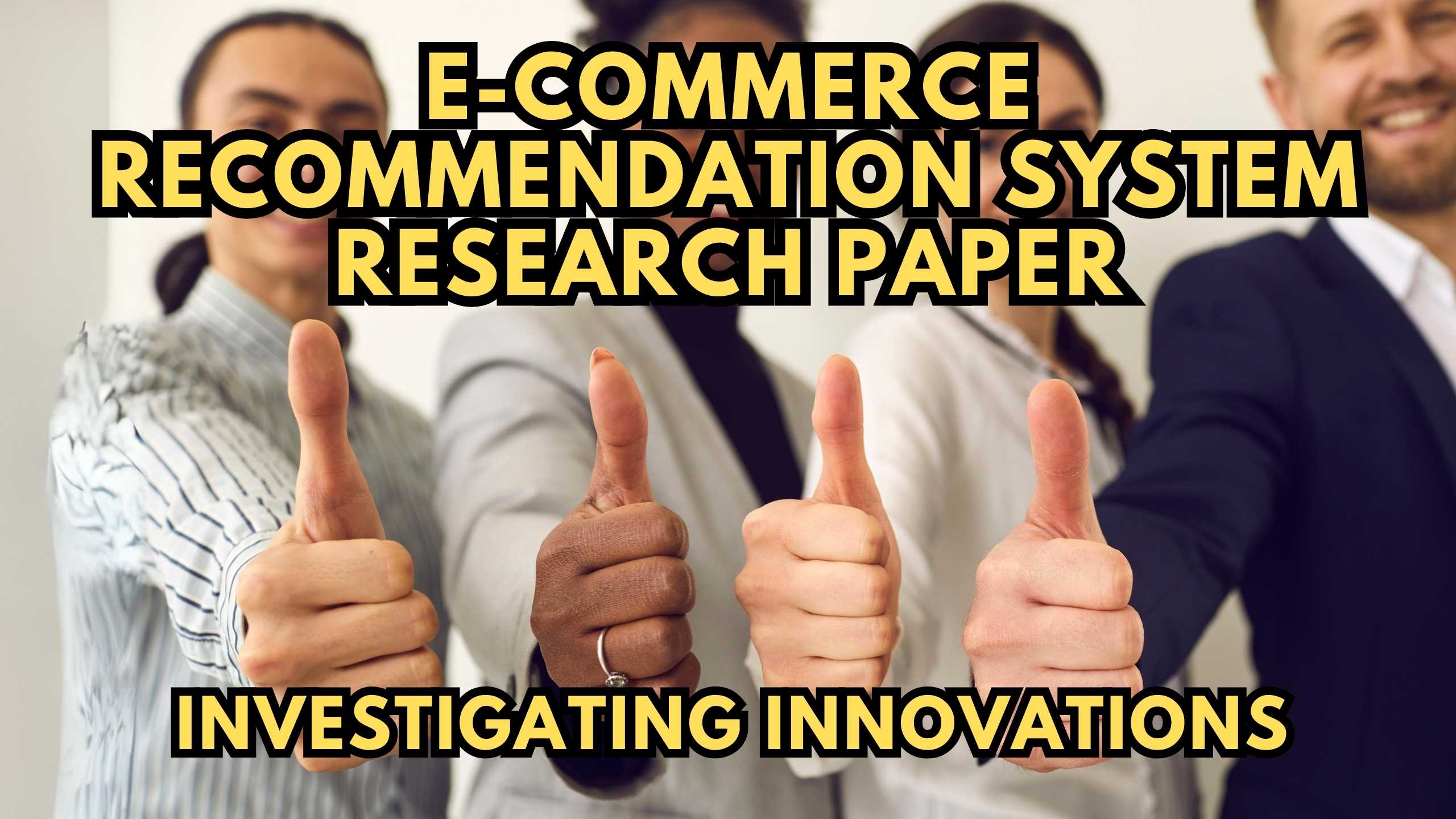
E-commerce Recommendation System Research Paper: Investigating Innovations
In the ever-evolving realm of e-commerce, recommendation systems play a crucial role in enhancing user experience and driving sales. This research paper explores the latest innovations in e-commerce recommendation systems, shedding light on advancements that redefine personalized shopping experiences.
1. Introduction to E-commerce Recommendation Systems
E-commerce recommendation systems have become the backbone of online retail, leveraging algorithms to analyze user behavior and provide personalized product suggestions. Innovations in machine learning, such as Amazon Personalize, are transforming the landscape by enabling businesses to create sophisticated recommendation models tailored to individual preferences. This level of personalization not only increases customer satisfaction but also contributes significantly to the overall revenue growth of e-commerce businesses.
Moreover, these systems go beyond merely suggesting products based on past behavior. Advanced recommendation engines, like those powered by Amazon Personalize, incorporate real-time data, current trends, and even external factors such as weather or events, ensuring that recommendations remain relevant and timely.
2. Deep Learning in Recommendation Systems
The integration of deep learning techniques in recommendation systems marks a significant stride in providing more accurate and context-aware suggestions. Platforms like TensorFlow Recommenders empower e-commerce businesses to leverage the power of deep learning for more nuanced and effective product recommendations, ultimately driving higher conversion rates. Deep learning enables systems to understand intricate patterns and relationships within vast datasets, resulting in recommendations that align closely with the user’s preferences and behaviors.
Furthermore, the continuous improvement in deep learning algorithms ensures adaptability to changing consumer behaviors. This adaptability is crucial in the dynamic e-commerce landscape, where consumer preferences can shift rapidly. TensorFlow Recommenders, for instance, provides a versatile framework that allows businesses to stay ahead by incorporating the latest advancements in deep learning.
3. Real-time Personalization
As users navigate through e-commerce platforms, real-time personalization has emerged as a game-changer. Systems like Dynamic Yield utilize real-time data to adapt recommendations instantly, ensuring that users encounter relevant suggestions based on their current browsing behavior. Real-time personalization not only enhances the user experience but also contributes significantly to the likelihood of conversion.
Furthermore, platforms like Dynamic Yield employ machine learning algorithms to analyze the behavior of users in real-time, enabling businesses to react promptly to changing preferences. By providing users with immediate and context-aware recommendations, e-commerce companies can create a sense of urgency and increase the chances of successful transactions.
4. Cross-channel Recommendation Integration
Innovations in e-commerce recommendation systems now extend beyond individual platforms. Integrating recommendations seamlessly across various channels, both online and offline, is a trend facilitated by solutions like Sailthru. This approach ensures a consistent and personalized experience for users, regardless of their interaction points with the brand. Sailthru, for instance, not only caters to online interactions but also integrates with in-store experiences, creating a unified and personalized journey for the customer.
Moreover, cross-channel integration is not limited to recommendation delivery but extends to the collection of data from multiple touchpoints. This holistic approach enables recommendation systems to have a more comprehensive understanding of user preferences and behaviors, resulting in more accurate and impactful suggestions.
5. Explainable AI in Recommendations
Understanding the rationale behind recommendations is gaining prominence. Explainable AI, as seen in platforms like LIME, allows e-commerce businesses to provide users with transparent insights into why certain products are suggested. This not only builds trust but also allows for fine-tuning recommendations based on user feedback. In the competitive e-commerce landscape, where user trust is paramount, being able to explain the reasoning behind recommendations fosters a sense of transparency and reliability.
Additionally, platforms like LIME provide businesses with the tools to interpret and communicate the outcomes of complex machine learning models. This interpretability is essential not only for gaining user trust but also for meeting regulatory requirements that demand transparency in algorithmic decision-making.
Relevant SaaS Products:
- Amazon Personalize: Harness the power of machine learning to create personalized recommendation models, redefining the way e-commerce businesses engage with their customers. Amazon Personalize’s adaptability and scalability suit businesses of all sizes, from startups to enterprise-level, making it an ideal solution.
- TensorFlow Recommenders: Implement deep learning techniques in recommendation systems for more accurate and context-aware product suggestions, driving higher conversion rates. TensorFlow Recommenders provides a flexible framework that allows businesses to experiment with and deploy state-of-the-art recommendation algorithms.
- Dynamic Yield: Utilize real-time personalization to adapt product recommendations instantly, providing users with relevant suggestions based on their current browsing behavior. Dynamic Yield’s robust platform empowers businesses to create dynamic experiences across various channels, increasing engagement and conversion rates.
- Sailthru: Seamlessly integrate cross-channel recommendations, ensuring a consistent and personalized user experience across various online and offline touchpoints. Sailthru’s comprehensive solution not only enhances recommendations but also facilitates a unified customer journey, driving brand loyalty.
- LIME: Implement explainable AI in recommendation systems, providing users with transparent insights into the rationale behind product suggestions and building trust. LIME’s interpretability tools enable businesses to understand and communicate the decisions made by complex machine learning models.
Conclusion
In conclusion, the continuous evolution of e-commerce recommendation systems is driven by innovations in machine learning, deep learning, real-time personalization, cross-channel integration, and explainable AI. As businesses strive to enhance user experiences and drive sales, staying abreast of these advancements is paramount. The ability to provide personalized, context-aware, and transparent recommendations not only meets the evolving expectations of consumers but also sets businesses apart in a highly competitive market.
Revolutionize Your E-commerce Strategy with Subscribed.fyi!
Ready to revolutionize your e-commerce strategy? Subscribed.fyi offers exclusive deals on essential SaaS tools. Sign up for free to access secret deals and unlock savings on recommendation system tools and other essential resources. Elevate your personalized shopping experiences and drive conversions with Subscribed.fyi.
Relevant Links: