Functioning of Personalized Recommendations: Insights
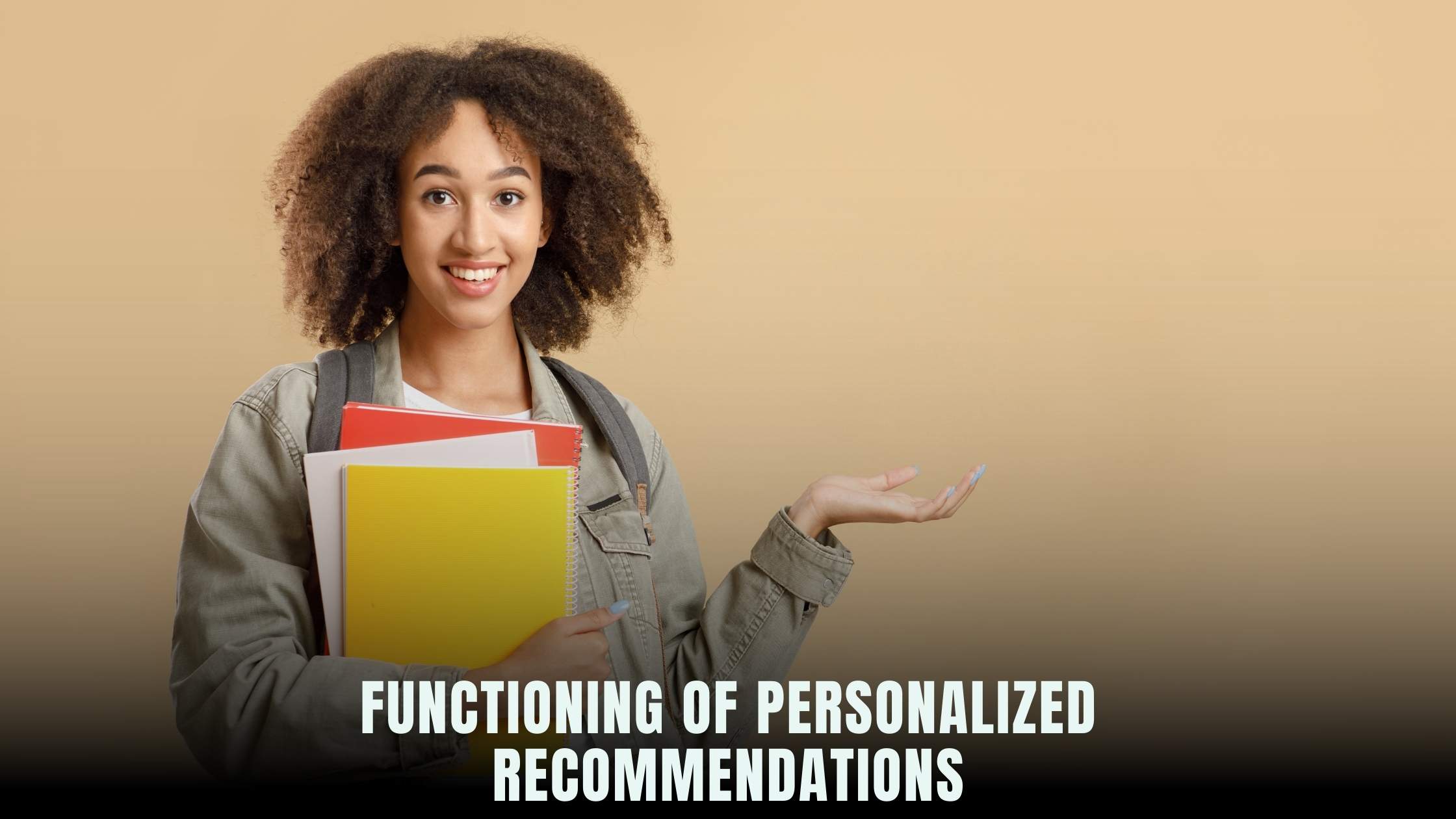
In today’s digital age, where information overload is the norm, personalized recommendations have become a crucial tool for businesses seeking to engage and retain customers. From e-commerce platforms to streaming services, personalized recommendations leverage data analytics and machine learning algorithms to deliver tailored content, products, and experiences to users based on their preferences, behavior, and past interactions. In this article, we’ll delve into the functioning of personalized recommendations, explore the underlying technologies and methodologies, and discuss their significance in driving customer satisfaction and loyalty.
Understanding How Personalized Recommendations Work
Personalized recommendations rely on sophisticated algorithms and data analysis techniques to analyze user data and generate relevant suggestions. The process typically involves the following steps:
- Data Collection: The first step in personalized recommendations is gathering user data from various sources, including browsing history, purchase behavior, demographic information, and explicit user preferences (e.g., ratings, likes, and dislikes).
- Data Processing: Once collected, the user data is processed and transformed into a structured format suitable for analysis. This may involve cleaning the data, removing duplicates or outliers, and organizing it into relevant categories or attributes.
- Algorithm Selection: Next, appropriate algorithms are selected based on the type of recommendations needed (e.g., content-based, collaborative filtering, or hybrid approaches). These algorithms analyze the user data to identify patterns, similarities, and relationships between users, items, and interactions.
- Feature Engineering: In this step, additional features or attributes may be engineered from the raw data to improve the performance of the recommendation algorithms. For example, user profiles may be enriched with demographic information or past purchase history to enhance the accuracy of the recommendations.
- Model Training: The selected algorithms are trained using historical data to learn patterns and relationships between users and items. Machine learning techniques such as regression, classification, or clustering may be used to train the recommendation models.
- Recommendation Generation: Once trained, the recommendation models generate personalized suggestions for users based on their profiles and preferences. These recommendations may take the form of product recommendations, content recommendations, personalized playlists, or targeted advertisements.
- Feedback Loop: Finally, the system collects feedback from users on the recommended items (e.g., clicks, purchases, ratings) to evaluate the effectiveness of the recommendations. This feedback is used to refine and improve the recommendation models over time, ensuring that they remain accurate and relevant.
Relevant SaaS Products for Personalized Recommendations
- Amazon Personalize: Amazon Personalize is a machine learning service that enables businesses to create personalized product recommendations for their customers. With Amazon Personalize, businesses can deliver personalized experiences across websites, mobile apps, and email campaigns, driving engagement and conversions.
- Sailthru: Sailthru is a customer retention platform that offers personalized marketing solutions, including product recommendations, content personalization, and predictive analytics. With Sailthru, businesses can deliver relevant and timely messages to their customers, increasing loyalty and lifetime value.
- Dynamic Yield: Dynamic Yield is a personalization platform that helps businesses optimize customer experiences through real-time personalization, product recommendations, and A/B testing. With Dynamic Yield, businesses can deliver tailored content and offers to each customer segment, driving engagement and conversions.
- LimeSpot: LimeSpot is an AI-powered personalization platform designed for e-commerce businesses. With LimeSpot, businesses can create personalized product recommendations, upsell and cross-sell suggestions, and targeted promotions to increase sales and customer satisfaction.
- Barilliance: Barilliance is an e-commerce personalization platform that offers solutions for product recommendations, behavioral targeting, and cart abandonment recovery. With Barilliance, businesses can deliver personalized experiences across multiple channels, driving conversions and revenue.
Leveraging Subscribed.FYI Deals for Personalized Recommendations Solutions
For businesses looking to enhance their personalized recommendation capabilities, Subscribed.FYI offers a curated selection of SaaS products that provide advanced personalization tools and resources. From machine learning-powered recommendation engines to customer segmentation and targeting solutions, Subscribed.FYI Deals provides access to innovative technologies that can help businesses deliver personalized experiences and drive customer engagement and loyalty.
Relevant Product Links: