Techniques for Product Recommendation: Exploring Options
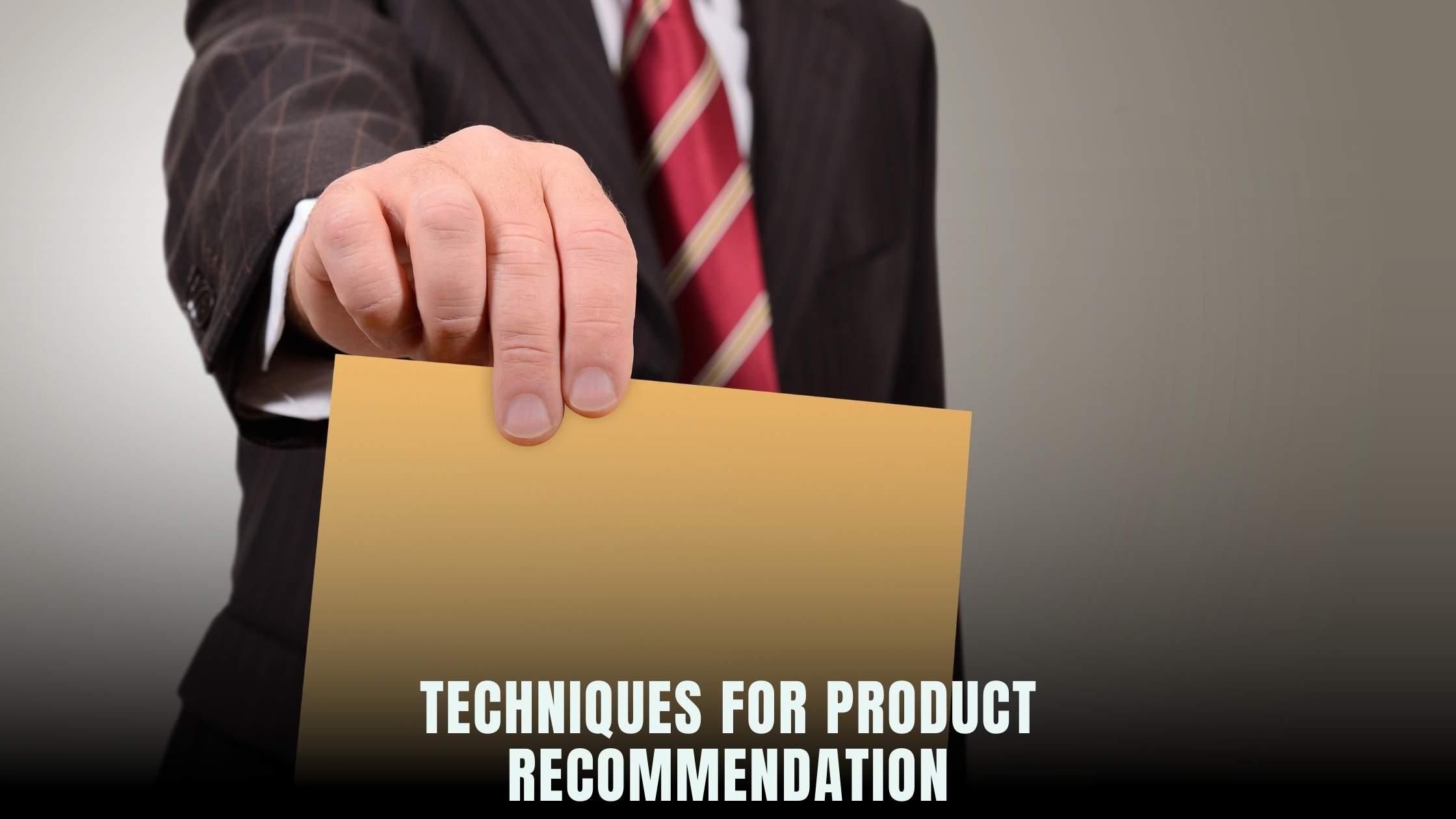
In today’s digital age, product recommendation plays a crucial role in enhancing the shopping experience for consumers and driving sales for businesses. From personalized suggestions to algorithmic recommendations, there are various techniques employed to guide consumers towards products that align with their preferences and interests. In this article, we’ll delve into the different techniques for product recommendation, exploring options that businesses can leverage to optimize their e-commerce strategies and delight their customers.
Understanding Product Recommendation Techniques
Product recommendation techniques utilize data analysis, machine learning algorithms, and user behavior patterns to suggest relevant products to consumers. Let’s explore some of the common techniques used in product recommendation:
1. Collaborative Filtering
Collaborative filtering analyzes user behavior and preferences to generate recommendations based on similarities between users. This technique identifies patterns in user interactions, such as purchase history, ratings, and preferences, to recommend products that similar users have liked or purchased. Collaborative filtering can be implemented using user-based or item-based approaches, depending on the nature of the recommendation system.
2. Content-Based Filtering
Content-based filtering recommends products based on their attributes and features, focusing on the similarity between items rather than user behavior. This technique analyzes product descriptions, attributes, and metadata to identify items that match a user’s preferences. Content-based filtering is particularly effective for recommending products with specific characteristics or qualities that align with a user’s interests.
3. Hybrid Recommendation Systems
Hybrid recommendation systems combine multiple techniques, such as collaborative filtering and content-based filtering, to generate personalized recommendations. By leveraging the strengths of different approaches, hybrid systems can overcome limitations and improve the accuracy of recommendations. These systems offer flexibility and adaptability, allowing businesses to tailor recommendations based on user preferences and behavior.
4. Association Rule Mining
Association rule mining identifies relationships and patterns between items in transactional data to generate recommendations. This technique analyzes historical purchase data to identify frequent itemsets and association rules, such as “customers who bought X also bought Y.” By uncovering implicit relationships between products, association rule mining can generate relevant recommendations and encourage cross-selling and upselling opportunities.
5. Reinforcement Learning
Reinforcement learning utilizes feedback mechanisms to optimize product recommendations over time. This technique learns from user interactions and adjusts recommendations based on user feedback and outcomes. Reinforcement learning algorithms aim to maximize long-term rewards, such as user engagement or conversion rates, by dynamically adapting recommendation strategies to evolving user preferences and market dynamics.
Relevant SaaS Products for Product Recommendation
- Amazon Personalize: Amazon Personalize is a machine learning service that enables businesses to create personalized product recommendations for their customers. By leveraging advanced algorithms and data analytics, Amazon Personalize delivers tailored recommendations based on user behavior and preferences.
- SAP Customer Experience: SAP Customer Experience offers a suite of solutions, including SAP Marketing Cloud, that enable businesses to deliver personalized product recommendations across various channels. With features such as predictive analytics and machine learning, SAP Customer Experience helps businesses optimize their marketing strategies and drive customer engagement.
- Salesforce Commerce Cloud: Salesforce Commerce Cloud provides AI-powered product recommendation capabilities to help businesses deliver personalized shopping experiences. By analyzing customer data and behavior, Salesforce Commerce Cloud recommends relevant products to shoppers, increasing conversion rates and driving revenue growth.
- Google Recommendations AI: Google Recommendations AI is a machine learning solution that enables businesses to deliver personalized product recommendations at scale. By analyzing user interactions and preferences, Google Recommendations AI helps businesses enhance the shopping experience and drive customer loyalty.
- Dynamic Yield: Dynamic Yield is a personalization platform that offers product recommendation solutions to e-commerce businesses. With features such as real-time targeting and predictive algorithms, Dynamic Yield empowers businesses to deliver tailored product recommendations across web and mobile channels.
Leveraging Subscribed.FYI Deals for Product Recommendation Solutions
For businesses seeking to enhance their product recommendation capabilities and drive conversions, Subscribed.FYI offers exclusive deals and insights on top SaaS products. From AI-powered recommendation engines to personalization platforms, Subscribed.FYI Deals provides access to innovative solutions that can help businesses optimize their e-commerce strategies and deliver compelling product recommendations to their customers.
Relevant Product Links:
- Amazon Personalize
- SAP Customer Experience
- Salesforce Commerce Cloud
- Google Recommendations AI
- Dynamic Yield
- Subscribed.FYI
- Subscribed.FYI Deals