Implementing Recommendation Engines: Best Practices
- AI Writing Assistant Popular Tools AI Tools
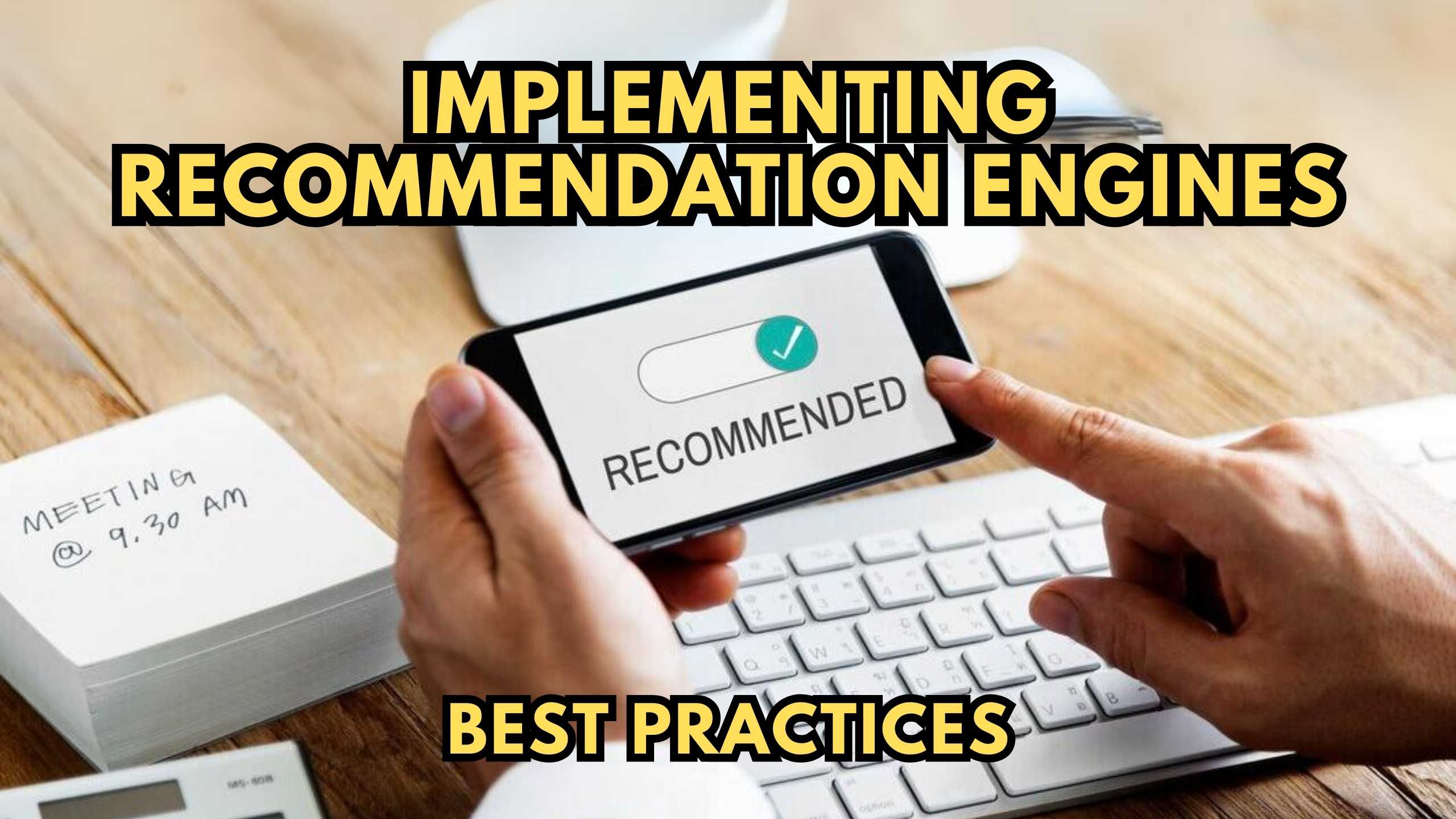
Implementing Recommendation Engines: Best Practices
Recommendation engines have become integral in delivering personalized user experiences across various platforms. In this blog, we will explore the best practices for implementing recommendation engines, shedding light on key considerations, strategies, and the role of relevant SaaS products in optimizing this process.
1. Understanding User Data
Implementing a recommendation engine starts with a deep understanding of user data. Tools like Mixpanel allow businesses to track user interactions, preferences, and behaviors, providing a robust foundation for creating personalized recommendations. Utilizing such tools ensures that your recommendation engine is built on accurate and insightful data.
2. Choosing the Right Algorithm
Selecting the appropriate recommendation algorithm is crucial for the success of your engine. Platforms like Google Cloud AI Platform offer a range of machine learning tools and algorithms. Leveraging these resources ensures that your recommendation engine tailors suggestions effectively based on user behavior, enhancing the overall user experience.
3. Real-time Updates
To keep recommendations relevant, real-time updates are essential. SaaS products like Segment facilitate the integration of real-time data, ensuring that your recommendation engine adapts to changes in user behavior promptly. This dynamic approach guarantees that users receive suggestions that align with their current preferences.
4. Implementing A/B Testing
Optimizing your recommendation engine requires continuous improvement. Tools like Optimizely enable A/B testing, allowing you to experiment with different algorithms and parameters. This iterative refinement ensures that your recommendation engine evolves, delivering increasingly accurate and valuable suggestions over time.
5. Ensuring Scalability
Scalability is a critical consideration, especially as user bases expand. SaaS products like Amazon Personalize provide scalable solutions for recommendation engines. By utilizing these services, businesses can ensure that their recommendation systems can handle growing amounts of data and user interactions without compromising performance.
Recommended SaaS Products:
- Mixpanel: Lay the foundation for your recommendation engine with accurate user data tracking, enhancing personalization efforts.
- Google Cloud AI Platform: Choose from a variety of machine learning tools and algorithms to tailor recommendations effectively based on user behavior.
- Segment: Keep your recommendations dynamic with real-time data integration, ensuring suggestions align with current user preferences.
- Optimizely: Implement A/B testing for iterative refinement, optimizing your recommendation engine for accuracy and value.
- Amazon Personalize: Ensure scalability for your recommendation engine, accommodating growing data and user demands seamlessly.
Conclusion
In conclusion, successful implementation of recommendation engines requires a strategic approach that encompasses user data understanding, algorithm selection, real-time updates, A/B testing, and scalability considerations. By adhering to these best practices and leveraging relevant SaaS products, businesses can create recommendation engines that not only enhance user experiences but also adapt and evolve to meet changing demands.
Revolutionize Personalization with Subscribed.fyi!
Ready to take your recommendation engine to the next level? Subscribed.fyi offers exclusive deals on essential SaaS tools. Sign up for free to unlock secret deals and access savings on Mixpanel, Google Cloud AI Platform, Segment, Optimizely, and Amazon Personalize. Elevate personalization in your recommendation engine and stay ahead in the competitive landscape with Subscribed.fyi.
Relevant Links: