Machine Learning in Healthcare: Current Applications and Future Prospects
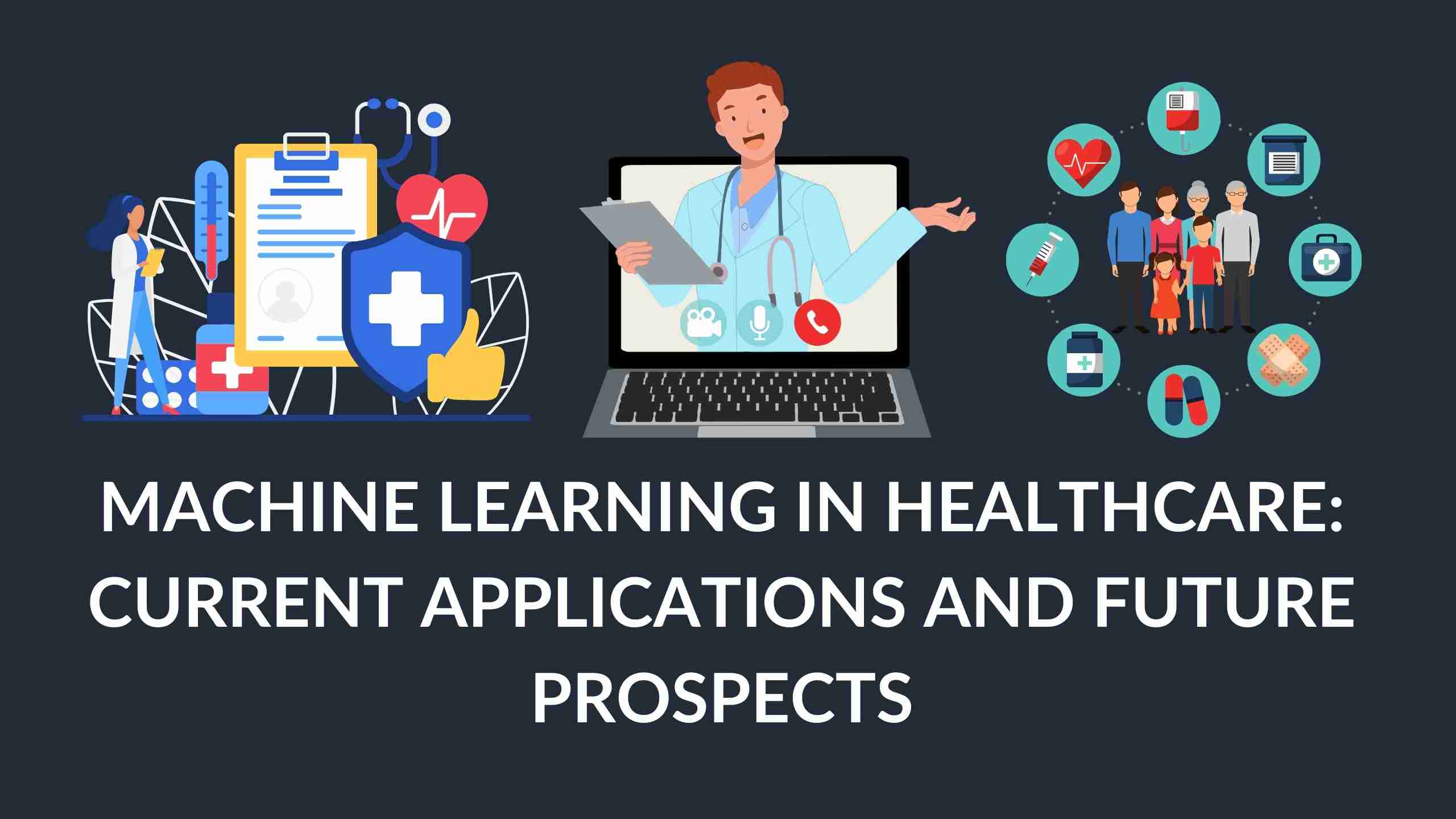
Machine Learning in Healthcare: Current Applications and Future Prospects
In the ever-evolving landscape of healthcare, the integration of machine learning (ML) has emerged as a transformative force. From enhancing diagnostics to optimizing treatment plans, machine learning applications in healthcare are diverse and hold immense promise. This article explores the current applications of machine learning in the healthcare industry and envisions the future prospects it brings.
Understanding Machine Learning in Healthcare
Defining Machine Learning
Machine learning involves the use of algorithms and statistical models to enable computer systems to perform tasks without explicit programming. In healthcare, these algorithms analyze data, identify patterns, and generate insights to support clinical decision-making.
Current Applications in Healthcare
1. Diagnostics and Imaging
Machine learning algorithms enhance diagnostic accuracy by analyzing medical images. From detecting abnormalities in X-rays to identifying early signs of diseases in MRIs, ML contributes to faster and more precise diagnostics.
2. Personalized Treatment Plans
Machine learning analyzes patient data to develop personalized treatment plans. By considering individual variations in genetics, lifestyle, and response to treatment, ML helps optimize therapeutic interventions for better outcomes.
3. Predictive Analytics for Patient Outcomes
Predictive analytics powered by machine learning models forecast patient outcomes. These models analyze historical data to identify patients at risk of specific conditions, allowing for proactive and preventive interventions.
Future Prospects of Machine Learning in Healthcare
1. Drug Discovery and Development
Machine learning accelerates drug discovery by analyzing complex biological data. ML algorithms identify potential drug candidates, predict their efficacy, and streamline the drug development process.
2. Remote Patient Monitoring
The future holds advancements in remote patient monitoring through wearable devices and sensors. Machine learning algorithms will analyze continuous streams of data, providing real-time insights into a patient’s health and facilitating early intervention.
3. Natural Language Processing for Clinical Documentation
Natural Language Processing (NLP) powered by machine learning simplifies clinical documentation. Automated analysis of medical notes and reports improves accuracy, reduces administrative burden, and enhances overall efficiency.
4. Genomic Medicine
Machine learning plays a pivotal role in deciphering genomic data. ML algorithms analyze genetic information to identify patterns associated with diseases, enabling personalized treatment strategies based on an individual’s genetic makeup.
Ethical Considerations and Privacy Concerns
While the integration of machine learning in healthcare brings unprecedented advancements, it also raises ethical considerations and privacy concerns. Striking a balance between harnessing the power of data and safeguarding patient privacy is imperative for the responsible implementation of ML in healthcare.
Conclusion
Machine learning has already made significant strides in revolutionizing healthcare, from improving diagnostics to enabling personalized medicine. As technology continues to evolve, the future prospects of ML in healthcare hold the promise of more efficient drug discovery, enhanced remote patient monitoring, streamlined clinical documentation, and personalized genomic medicine. However, ethical considerations must guide the responsible implementation of these technologies to ensure the highest standards of patient care and data security.